“They are taking pictures of taking pictures.” — Don DeLillo, White Noise.
Before we named them photographs, we had different proposals: Photogenes, Heliographs, Photograms, Sun-prints. None of them caught on, but each one proposes a unique perspective of how people made sense of them.
Photogene, for example, refers to the after-image burned onto the human retina just after closing our eyes. It’s a beautiful way of imagining the relationship of film and camera. In 1859, Rochester, NY resident E. Peshine Smith offered photograms — merging “light” and “telegram” to suggest a tool for sending light from one place to another.
Following that Rochester tradition of failed naming schemes, I have my own proposal for what to call AI generated images. It won’t succeed, but I offer it up in the spirit of poetic techno-social marginalia.
AI Photography is not Photography
Before make my suggestion, let’s talk about Photograph. It’s a blending of “light” (photo) which, through exposure to film or a sensor, is inscribed or “written” (graphy) into image form: the photograph.
AI images are not photographs. AI images use light differently. Through pixels, digital information is an instruction for a screen: they produce light, rather than capture it. This presence to light gave photographs their original documentary power: light was a stamp that certified the camera’s presence to whatever was photographed. Digital images captured light on a sensor instead of film, but reproduced that light as images through pixels on screens.
AI images are not “present” to anything. So what do AI images “write” with?
We might say they write with data. Arguably, that’s the essence of what AI images draw from and with. But data isn’t useful in isolation. You have to organize data, move it around, for it to become something else. In GANs, data comes from specific datasets, and the images it produces have to be tested against itself to produce something new.
With CLIP-based Diffusion models like DALLE2, data must be paired with categories. These categories make it possible to type an image into being. Categories shape what is “written” into an AI image, as does whatever data is present in the dataset. Data must be organized into categories, expressed into images through labels.
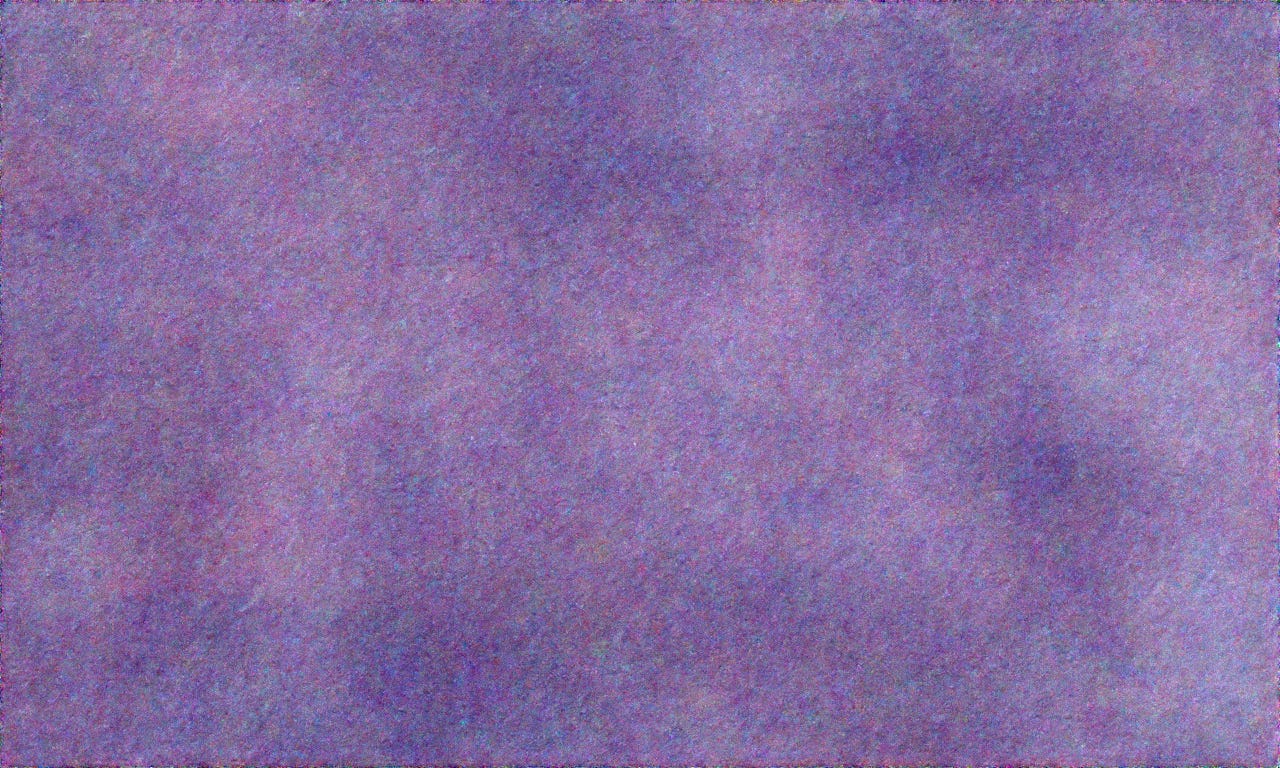
Diffusion starts from noise: the image above is a starting point for Disco Diffusion. It generates images from this noise based on the words you type. Your words steer the Diffusion model to “look for” certain values in that noise, sharpening the noise into an image with each pass.
Type in “The most photographed barn in America” and Stable Diffusion looks at each word, and the relationships of each of those words, to turn the above noise into some concept of “The most photographed barn in America.”
The Most Photographed Barn in America is not initially present in that purple fuzz. Instead, the model starts from that noise to seek out constellations of pixels that might recreate the associations it has learned from the dataset. Once the images in a dataset have informed a model, it’s not referencing those images anymore. It draws from representations of the categories of that dataset, prompted by words.
It isn’t “writing light” at all. It’s inscribing ontologies onto noise.
Great American Folk Ontology
That word, ontology, has never made anything clearer to anybody. Let’s break it down.
AI image tools create information from abstract representations of information. There is no light, no sensor, no film, no subject, just a mass of visual information which was translated into a system of categories. This system of categories is what makes the image you get when you type a prompt. This is literal: the words you type are paired to representations of those words.
Consider a library’s Dewey Decimal system. You could go to a bookshelf and scan the books, or go to the cards and scan through titles. If you scan through the titles, you might be able to produce an image in your mind — or a concept, if images aren’t how you think — that says “this cookbook and this cookbook are side by side, because they are both cookbooks, and French cuisine and German cuisine are next to each other because of alphabetical order.”
You can envision a bookshelf with that information. You don’t have to go to the bookshelf to see how the bookshelf is arranged, because you have a system of notes that represent the bookshelf. A bookshelf is organized by a particular logic reflecting biases: alphabetical order is a bias, yes, but perhaps distinctions between “world cuisine” from “American Cookbooks.”
Likewise, prompts are steered through categories, which creates the latent space, or space of possibility, from which images emerge. Asking for “The most photographed barn in America” is asking for a series of categories: most photographed, barn, in America. It also happens that “The Most Photographed Barn in America” is a real place, the Thomas Moulton Barn in Wyoming, which bares only a passing resemblance to Stable Diffusion’s interpretation.
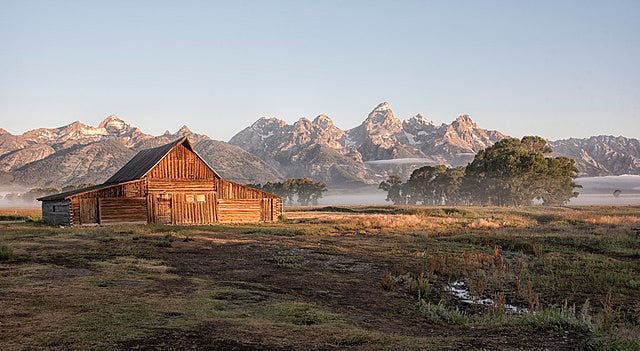
In information science, an ontology is:
“a representation, formal naming, and definition of the categories, properties, and relations between the concepts, data, and entities that substantiate one, many, or all domains of discourse. More simply, an ontology is a way of showing the properties of a subject area and how they are related, by defining a set of concepts and categories that represent the subject.” (Wikipedia)
This is also fair definition of what is written into an AI image.
These categories come from CLIP: the pairing of image captions to abstracted image data. These captions are written by people — quite often, I should note, human click-workers who are paid pennies for every label.
I’ve written plenty about the image datasets that go into these models, and how social systems shape the content of those datasets. CLIP is an additional layer, where humans make sense of images and inscribe that sense into the model.
“The Most Photographed Barn in America” prompt doesn’t give us images of the Thomas Moulton Barn, the way it might if, say, Diffusion was a search engine, or if that particular barn was significantly overrepresented in an image dataset. Instead, the model draws from its own ontology — category spaces, assigned by words, to describe underlying information patterns.
Ontolography: A Modest Proposal
As a name for AI images, I know that Ontolography will never catch on. Nobody likes the word ontology, or wants to see it more often. Even if they did, there are just too many O’s. (And all photographs are ontolographs.)
But this portmanteau of ontologic+graphy is worth a footnote. There are a lot of ways to define an ontology, but I’ll just go back to Wikipedia, which gives us this:
What ontologies in both computer science and philosophy have in common is the attempt to represent entities, ideas and events, with all their interdependent properties and relations, according to a system of categories.”
The output of AI image models are a completion of what Vilem Flusser noted as the movement of social activity into the image. For Flusser, the image was initially documentation of what was observed, but quickly dominated what was observed, so that social activity was increasingly organized for documentation as images. The camera moved from documentary tool to an organizing principle.
Ontolography, then, suggests a system of calling forth images without any direct observation or documentation at all. It’s all built on past documentation, like a photographer who only takes photographs of magazines. It’s an acknowledgement that we don’t need to do the things we want to document. We’ve done every pose enough that a machine could produce it, and now we don’t need to pose but only to reference past poses from our imagination and call them up.
The images that rise up from this movement are “pictures of pictures,” to steal DeLillo’s phrase. We don’t need events, we just need event categories. We can create documentation of a birthday party without eating a single piece of cake.
Ontolographs are images made from information categories instead of events.
We might cut an unwieldy O. Onto in Greek, “to be/that which is,” gives us ontic, “related to the existence of structure,” so we might do “ontograph” or “ontigraph.” Squint enough and it becomes “that which is structured is written.” (It may also be time to retire our reliance on Greek and Roman).
Ontographs exist, but barely. Tobias Kuhn coined the term in 2013 to describe charts of relationships within specific social systems: the example he provides is, quite honestly, a good simplification of how language works through CLIP and Diffusion, even if that’s far removed from its intention.
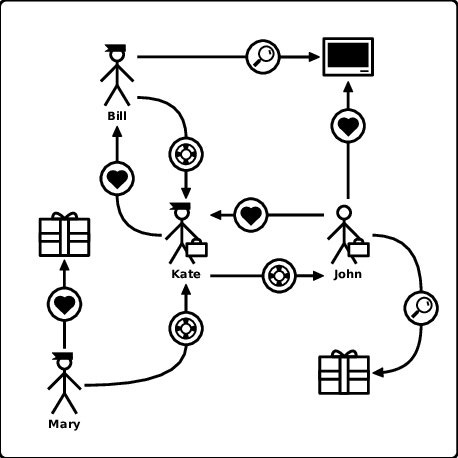
Regardless: The Case for Ontolography
Acknowledging that this name is doomed, I’ll offer up my case anyway. \
Ontolography moves AI images more precisely into the territory of ontology, emphasizing the domain-specific knowledge of the models that inform their production rather than confusing these pictures as representations of reality. They are shaped by the category assignments and labels we give them: change the data or the labels and you change the model, change the model and you change the resulting images.
Each training set creates a closed world of information through these labels, its own ontological system of meaning. The images are infographics of these structural arrangements.
There is no photo, because there is no light. There can be no recording of light in an AI image because there is no apparatus present for events. They produce light: literally, they inscribe ontologies into pixels. These ontologies are crafted from images without knowledge of the events that produced them.
The resulting image is written not from light but as a sequence of pixels informed by that data-limited world. Images and text intersect as information and then as a set of instructions: the Ontolograph is not light etching films or sensors, but the result of abstract categories that activates pixels. This is the work of ontology, what Husserl calls “the science of essences.” By that, we might understand the AI generated image as the writing of essences.
The term “Ontolography” reminds us not to look to the images but the structures that compose them: the systems that make the picture, rather than the light they produce.
Please share, circulate, quote, link, or reduce this post to its essence in order to regenerate endless variations. At the very least, it would be great to tell a friend about it: word of mouth means a lot to me.